Part 3: Replying to the Response to Our Manuscript Clarification of Aubry et al. 2018
Earlier this year, Aubry et al. published a paper in Journal of Strength and Conditioning Research using Stryd Pioneer entitled “An assessment of running power as a training metric for elite and recreational runners” (Aubry et al., 2018).
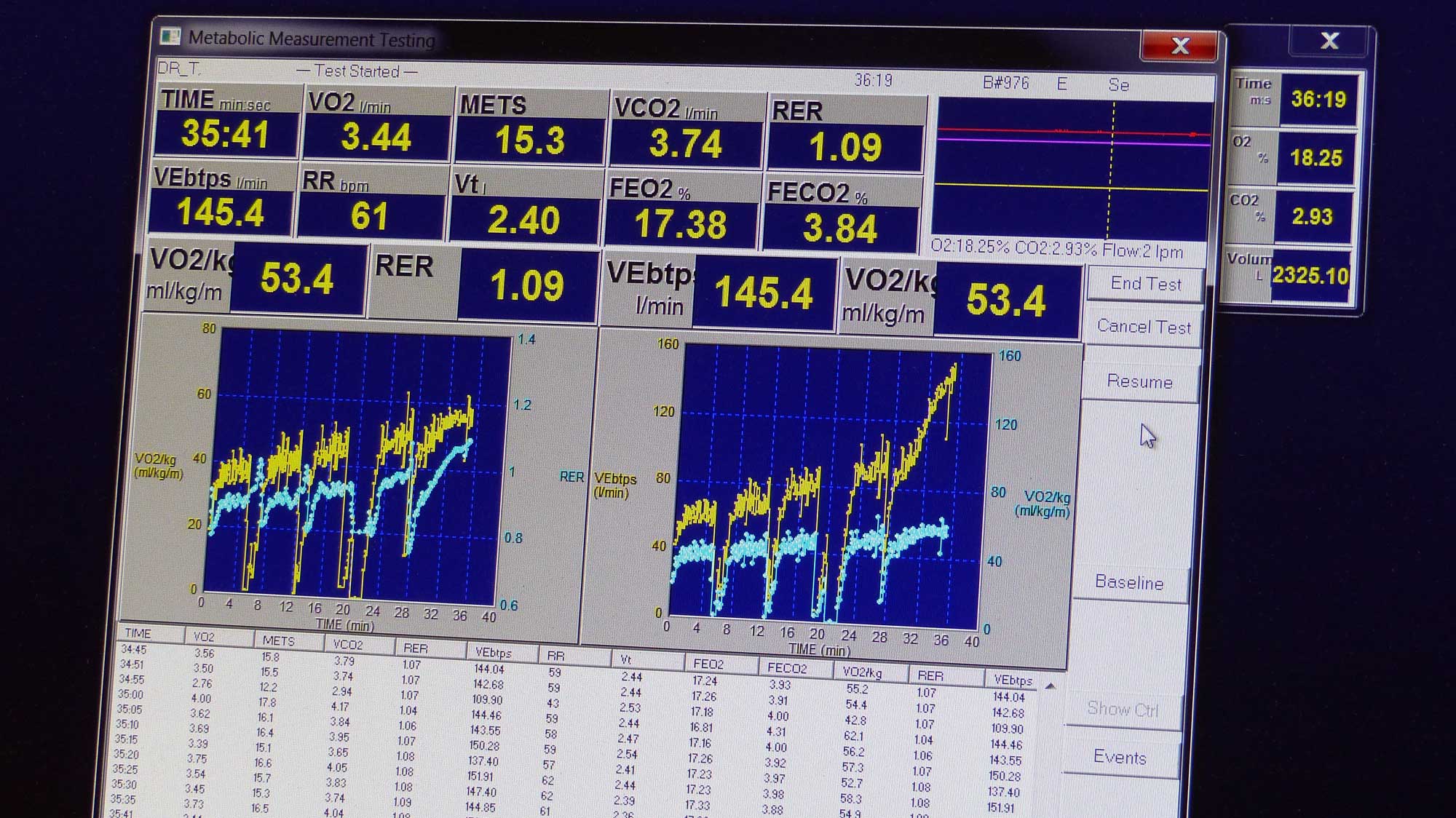
Note: Independent academic research helps to drive innovation. At Stryd, we are researchers, first and foremost. It’s always exciting to us to read about and learn from new testing of sports technology innovations by the wider research community. However, we are responding in detail now to research that we believe needs correcting regarding proper mathematical and physiological analyses. Science is self-correcting, and we believe that taking this opportunity to respond is an important and integral part of the evolution of sports technology.
Please read the post below. We encourage you to join in the discussion.
- Part 1: New Research Data Shows Stryd Pioneer Power Correlates with Metabolic Expenditure (June 2018)
- Part 2: Using appropriate mathematical and physiological analyses in Aubry et al. 2018 (December 2018)
- Part 3: Replying to the Response to Our Manuscript Clarification of Aubry et al. 2018 (December 2018)
Earlier this year, Aubry et al. published a paper in Journal of Strength and Conditioning Research using Stryd Pioneer entitled “An assessment of running power as a training metric for elite and recreational runners” (Aubry et al., 2018).
Manuscript Clarification: Methodological Flaws in Aubry, RL, Power, GA, and Burr, JF. An Assessment of Running Power as a Training Metric for Elite and Recreational Runners (December 2018)
Author Response to the Manuscript Clarification: Author Response (December 2018)
They normalized metabolic rate by speed to get cost of transport, performed a correlation analysis, and came to the conclusion "Running power is not sufficiently accurate as a surrogate of metabolic demand." Unfortunately, when the authors tweeted the paper out, the conclusion from this paper made the rounds on the internet. As often occurs, this happened without the methods, which contain significant flaws, being properly vetted. In fact, their paper had what in academic science are called “fatal flaws,” methodological issues so profound that they fundamentally change the study’s conclusion. We first contacted numerous colleagues in academia with neutral opinions of Stryd to verify their assessment of the methodological flaws we found in the methodology. Without exception, they agreed that these were fatal flaws.
Wanting to respond through the proper channels with the journal, we approached the editors about how to appropriately bring light to the methodological problems with the article. At their suggestion, we subsequently wrote a manuscript clarification (MC) for the journal, again vetted by academics with no love for Stryd, which we submitted on June 29th, and, when substantial revisions were requested on July 20th, resubmitted in revised form on July 31st. Our MC was published with the authors’ response in late November. After reading the response to our MC, which contained two demonstrable falsehoods, we asked the editors about the possibility of responding again. They stated that the journal’s policy was only allow one MC and one response but encouraged us to continue the discussion. The editors we dealt with have been universally honest and helpful, and we appreciate their assistance in the process of getting the truth out there. Our purpose here is therefore to reply to their response to our MC
We had two initial concerns with the study’s methods:
- Data collection methods are not consistent across surfaces, making effective comparison across surfaces impossible.
- Data analysis uses inappropriate normalization that leads to minimal variability in “metabolic demand” (actually cost of transport), violating one of the fundamental assumptions necessary for calculating a correlation coefficient.
Data collection methods are not consistent across surfaces, making effective comparison across surfaces impossible.
The first concern was with the data collection methods for the treadmill trials. For these data, with only 2-minute trials and no rest, rate of oxygen consumption values were not guaranteed to reach steady state, nor were rate of oxygen consumption values allowed to return to resting between trials. This is in contrast to the metabolic data collection methods for the overground data, where the trials were aligned with common, accepted, guaranteed to reach steady-state practice with longer trials and rest in between. It is true, as the authors claim in their response to our MC, that it takes less time to reach steady state when going from one running speed to a faster speed than from rest to that speed, but nowhere in the paper do the authors explain how steady state was determined or when it was reached, just that they used the highest 30 second average. The authors in their response to our MC state that steady-state was reached, but they offer no quantitative data to back up this claim, nor do they state what methods they used to determine when steady-state was reached or if they were consistent across conditions. Given that the value of metabolic rate on average increases until steady state, taking the highest 30 second average is no guarantee that steady state was actually reached, especially if the highest average was the last average. A single value says nothing about the rate of change.
The authors also state that “We expect the design team is glad that the kinetics do not lag for such an extended period of time for if they did, the reflection of metabolic demand by an accelerometer/speed-based device such as Stryd would be off by a matter of minutes with each and every change in pace.” This statement is clearly the kind of logical fallacy known as a “straw man.” We do not claim, nor have we ever claimed, that Stryd gives a correlate for instantaneous rate of oxygen consumption, which is nigh on impossible to measure with sufficient precision, never mind validate, but for steady-state rate of oxygen consumption under given conditions. It is worthwhile here to note that cycling has roughly the same metabolic time dynamics as running, if anything taking a longer time to reach steady state (Carter et al., 2000), but power in cycling, often called work rate, has been long proven and accepted to be a useful correlate for steady-state rate of oxygen consumption (Ettema and Lorås, 2009). Efficiency (mechanical power/metabolic power) in cycling is calculated using steady-state metabolic data; there are 26 studies that do so included in Ettema and Lorås’ review, too many to cite individually here. As was the case for validation of cycling power as a correlate for metabolic cost, all of our rate of oxygen consumption validation data has been collected using sufficiently long trials and proper determination of time to steady state and has therefore, like cycling, only used steady-state rate of oxygen consumption values.
To reiterate, the issue with the data collection methods is with a lack of consistency across conditions, either in the time of data collection, the rest period, or, most of all, in the determination of when steady state was reached. Without these, the data cannot be guaranteed comparable across conditions.
Data analysis uses inappropriate normalization that leads to minimal variability in what the authors called “metabolic demand” (actually cost of transport), violating one of the fundamental assumptions necessary for calculating a correlation coefficient.
A thorough mathematical demonstration of how these flaws lead to a false conclusion can be found in our previous blog post. However, here we wish to reply to the response to our MC, particularly because it contains two falsehoods.
In their response to our MC, the authors state that “If we remove the influence of speed on two variables that are linearly related to speed, then the relationship of those variables should not differ greatly.’ This is not true if one or more of the variables is proportional to speed. It is shown not to be true for this case, and, in particular, not true given that rate of oxygen consumption is approximately proportional to running speed in recreational and elite athletes (Batliner et al., 2018). Again, a thorough mathematical demonstration of the untruth of this statement for these data can be found in our previous blog post, but we will do a short summation here.
What the authors call “metabolic demand’ in Figure 2 is actually typically called “metabolic cost” or “cost of transport.” The units for this variable in the axis label even have units of time in the numerator and the denominator, suggesting all the details of the methods might not have been properly vetted during the revision process. Normalizing rate of oxygen consumption by speed to get cost of transport is a typical practice in physiology; it was done in Margaria et al.’s seminal 1963 paper (Margaria et al., 1963) and has been done hundreds if not thousands of times since. However, a strong linear relationship would not be expected between cost of transport, which is virtually constant or shallowly quadratic within a given subject across speed (Margaria et al., 1963, Bramble and Lieberman, 2004, Steudel-Numbers and Wall-Scheffler, 2009), and any measure that is linearly dependent upon speed normalized by speed. If one of your variables does not have sufficient variation, it is mathematically impossible to find a significant correlation value indicating a linear relationship. Any correlation whatsoever between the normalized measures would be small and due to chance, unaccounted for nonlinearities, or subject variation, not the dominant linear relation with velocity that underlies both non-normalized measures.
The authors also claim in their response to our MC that “Irrespective of correlational data, we have addressed the changes in non-normalized “power” and VO2 in Table 2, and it is apparent that the changes observed in VO2 with increasing speed were not reflected in “power.”” This statement is demonstrably false. We had hoped to do a proper analysis of the non-normalized data, as using averaged group data leads to an overestimation of the correlation coefficient. Unfortunately, the authors declined to share their non-normalized individual subject data with us. Instead, we were forced to use their group mean data from Table 2, the only data presented in the paper that was not improperly normalized. In our previous blog post, we calculated R values for each group for each condition for these data and found them all to give values of 0.99. While these values are certainly larger than they would be for the individual data, they definitively show that the changes observed with increasing speed were linearly reflected in Stryd power, proving the authors’ previous statement about Table 2 to be untrue.
The authors in their response to our MC claim to have done the analysis in a non-normalized fashion and get R values of 0.3-0.5, but these data are never shown either in data or figure form, nor do these numbers fit with our analysis of their data nor our data. If the authors were to do the analysis using the correct units, rather than normalizing by velocity, and show their data, they would find a clear correlation between rate of oxygen consumption and Stryd power. If they did not, their calculations must be wrong. We would be happy to point out where, if they were to share their data. Further, Stryd Pioneer and its algorithms are far cruder than Stryd’s current capabilities. Performing this study with a current Stryd foot pod or our next update would lead to still higher correlation values.
References
AUBRY, R. L., POWER, G. A. & BURR, J. F. 2018. An assessment of running power as a training metric for elite and recreational runners. J Strength Cond Res, 32, 2258-2264.
BATLINER, M. E., KIPP, S., GRABOWSKI, A. M., KRAM, R. & BYRNES, W. C. 2018. Does metabolic rate increase linearly with running speed in all distance runners? Sports Medicine International Open, 2, E1-E8.
BRAMBLE, D. M. & LIEBERMAN, D. E. 2004. Endurance running and the evolution of Homo. Nature, 432, 345-52.
CARTER, H., JONES, A. M., BARSTOW, T. J., BURNLEY, M., WILLIAMS, C. A. & DOUST, J. H. 2000. Oxygen uptake kinetics in treadmill running and cycle ergometry: a comparison. J Appl Physiol, 89, 899-907.
ETTEMA, G. & LORÅS, H. W. J. E. J. O. A. P. 2009. Efficiency in cycling: a review. 106, 1-14.
MARGARIA, R., CERRETELLI, P., AGHEMO, P. & SASSI, G. 1963. Energy cost of running. 18, 367-370.
STEUDEL-NUMBERS, K. L. & WALL-SCHEFFLER, C. M. 2009. Optimal running speed and the evolution of hominin hunting strategies. Journal of Human Evolution, 56, 355-360.